Using advanced learning models to make wildlife monitoring less time consuming and more accurate
Monitoring the presence, distribution, group composition and behavior of wildlife is critical in helping asset operators demonstrate the preservation of the local environment. And it’s often a requirement to comply with government regulations and sustainability standards.
But it can be difficult because wild animals are hard to spot. And what may seem like a straightforward undertaking can be painstaking, time consuming, and expensive.
“Usually, our analysts sift through images captured by remote cameras using heat and motion triggers to create valuable reporting,” explains Erica Crowther, Environmental Scientist, Worley Consulting, our consulting business. “These programs produce millions of images per year, but a lot of time is wasted because more than 90 percent of images are discarded as false triggers.
“So, we connected with Worley’s data science team to explore whether we could apply machine learning to automate this process and in doing so, create a new approach to monitoring wildlife surrounding one of our customer’s energy assets.”
Automating wildlife monitoring in two steps
We began the project by developing an automated classification process with two key steps.
First, we used a transfer learning technique to train a learning classification model to group the images into two categories: false triggers (confirming no animal presence) and true positives (confirming the presence of an animal).
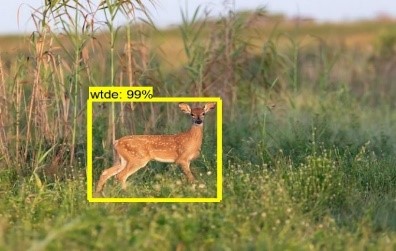
Next, we trained a learning object identification model to classify true positive images and organize these into multiple animal categories. With this model, wildlife is automatically identified and marked in the image. This helps the model reduce the number of false classifications and learn the features of the animal more accurately.
To date, the model has achieved an accuracy rate of 95 percent, enabling the Advisian team to deliver wildlife monitoring studies with greater efficiency and precision.
A proven solution with wide-reaching potential
Although this model was developed to support a specific customer need, its potential value is much broader. The model can be scaled to catalogue and classify different species in different habitats and environments around the world.
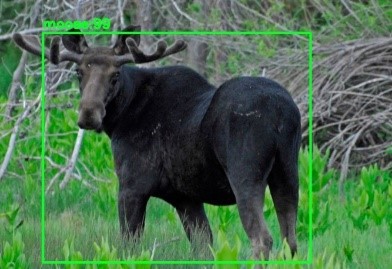
“The Advisian environmental team can build on this model with future customers, wherever their operations are around the world,” says Vishal Mehta, Senior Vice President, Information and Digital Delivery. “And it’s a great example of how digital can bring enormous value to the way we work and contribute towards creating more sustainable outcomes.”